Healthcare and the challenges of big data
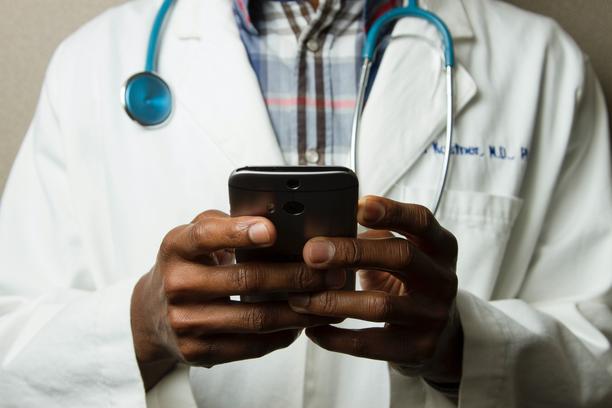
Globally, healthcare is at the centre of a big data boom that may prove to be one of the most significant drivers of healthcare change in the next decade. Today, we're collecting more information than at any point in healthcare history.
In the UK, big data strategy is spearheaded by Health Secretary Jeremy Hunt and NHS England. In the US it is led by the Obama Administration's big data R&D initiative.
American federal health agencies are contributing five-years of public datasets and analytics for genomics and molecular research to a cloud-based research platform hosted by BT. This will compliment the de-identified NHS population, medical and biological datasets that already reside in the cloud.
Failure to deliver
Analyzing these data is expected to enable earlier detection of effective treatments, better targeted clinical decisions, real-time bio-surveillance and accurate predictions of who is likely to get sick. These promises are predicated on the interoperability of the data and the availability data analysts and managers.
According to a 2011 McKinsey & Company Report, "The US alone faces a shortage of 140,000 to 190,000 people with deep analytical skills as well as 1.5 million managers and analysts to analyze big data and make decisions based on their findings".
So far, healthcare systems have failed to deliver on big data promises.
Big data's potential benefits for healthcare
Driving this new open epidemiology research initiative is big data's successes in other sectors and the pressing need to modernise healthcare infrastructure. Like many emerging technologies, the future of big data in healthcare is seen to be full of benefits.
Little data
The promises of big data overlook the challenges of little data. Little data collected at the unit level have two principal challenges: accuracy and completeness.
Insights from data are predicated upon the accuracy and completeness of that data. When data are systematically biased through either errors or omissions, any correlations made are unreliable and could result in misguided confidence or the misallocation of scarce resources.
In healthcare, important clinical data, such as symptoms, physical signs, outcomes and progress notes rely on human entry at the unit level. This is unlikely to change. Health professionals at the unit level will continue to exert discretion over their clinical documentation. Unit level information - little data - presents the biggest challenge for the interoperability within and among healthcare big data initiatives.
Ian Angel, an Emeritus Professor of Information Systems at the London School of Economics uses the North Staffordshire Hospital debacle to illustrate how professionals at the unit level react to data-driven ruled-based management by manipulating data. "Surgeons pushed dying patients out of the operating room into corridors to keep "death in surgery" figures low. Ambulances parked in holding-patterns outside overstretched A&E units to keep a government pledge that all patients be treated within four hours of admission".
Proprietary systems
Big data are most newsworthy, but least effective. Little data are most influential, but least newsworthy.
There are a plethora of technology vendors vying to help a plethora of health providers' to lock-in millions of patients at the unit level with proprietary software systems. Scant attention is given to this.
Further, software vendors predicate their sales on the functionality and format of their systems and data. This obscures the fact that the data format is 100% proprietary. Over time, this cycle of proprietarily locking-in patients at the unit level has created a sclerosis in healthcare infrastructures, which is challenging to unblock. This presents a significant challenge to interoperability and the success of big data.
Errors in little data
Little data documentation can be enabled by technology. For instance, machine learning is a form of artificial intelligence that trains systems to make predictions about certain characteristics of data. While machine learning has proved successful for identifying missing diagnoses, it is of limited use for symptoms and the findings of physical examinations.
Despite technological advances, clinicians' notes remain the richest source of patient data. These are largely beyond the reach of big data.
Another technology, which supports clinical documentation, is natural language processing (NLP). This identifies key data from clinical notes. However, until the quality of those notes improve, it will be challenging for NLP programmes to procure the most salient information. Continued investment in technical solutions will improve data accuracy, but without fundamental changes in how care is documented, technology will have limited ability to rid data of systematic errors.
Incomplete data
Even if we achieve perfect data accuracy, we're still faced with the challenge of data fragmentation. Incomplete data are common in clinical practice and reflect the fragmented nature of our healthcare systems. Patients see multiple health professionals who do not communicate optimally.
Incomplete data, like inaccurate data, can also lead to missed or spurious associations that can be wasteful or even harmful to patient care.
Privacy is less challenging
Solutions to address fragmented data are no easier than those to address inaccurate data. For decades policy makers have pursued greater interoperability between electronic clinical systems, but with little success.
Recent initiatives on interoperability of big data primarily focus on moving specific clinical data, such as laboratory test results, between discrete health providers. This does little to ensure that provider organizations have a comprehensive picture of a patient's care across all care sites.
Privacy advocates are understandably concerned about efforts to aggregate data. However, with adequate de-identification and security safeguards, the risks of aggregation can be minimized and the benefits of better care at lower costs are substantial.
Takeaway
To reap the benefits from big data requires that we understand and effectively address the challenges of little data. This is not easy. But ignoring the challenges is not an option.