A prescription for an AI inspired MedTech industry
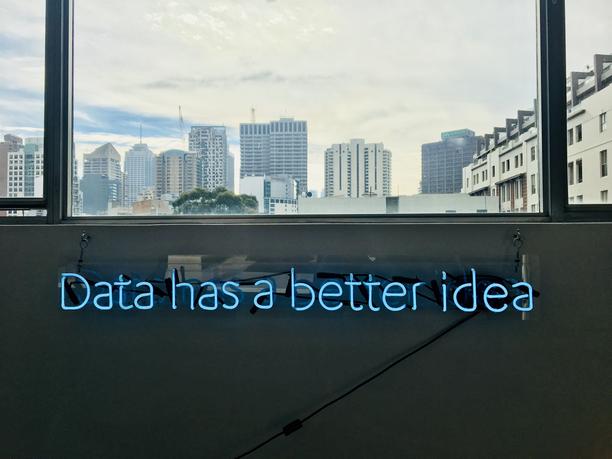
A prescription for an AI inspired MedTech industry
Thinking beyond physical products and the growing significance of AI in MedTech markets
A wind of change is blowing through MedTech markets, which has prompted some key opinion leaders to think beyond physical products and begin to use artificial intelligence (AI) techniques to develop value added services that bolt-on to their existing physical offerings to improve clinical care and economic efficiencies while providing access to new revenue streams. Bryan Hanson, Zimmer-Biomet’s CEO, recently suggested that >70% of his company’s R&D spend is now being invested in data informatics and robotics. Not far behind is Stryker, another global orthopaedic corporation, which has implemented AI strategies to improve care and differentiate its offerings. Both are thinking beyond their physical products to create a suite of services derived from AI enhanced data collected from their existing devices. Such actions provide a template that can be copied by other enterprises. How long will it take for AI solutions to represent a significant percentage of MedTechs’ revenues? In this Commentary
This Commentary: (i) describes the growing significance of AI, (ii) explains the difference between data mining, AI, and machine learning, (iii) illustrates AI technologies that have become an accepted part of our everyday lives, (iv) highlights technical drivers of AI solutions, (v) describes obstacles to the development of AI systems, (vi) indicates how such obstacles may be reduced, (vii) describes Zimmer’s and Stryker’s AI driven data initiatives, (viii) suggests that the Zimmer-Stryker AI template has broad potential, (ix) suggests that AI systems can breathe life into 'dead data', (x) provides an example of a company at the intersection of medical information and AI techniques, (xi) describes the origins of the phrase, ‘wind of change’, and defines the ‘winds’ driving change in current MedTech markets, (xii) reports that ~80% of B2B sales in the economy generally are digitally driven, (xiii) provides some reasons for MedTechs’ slow adoption of AI systems, (xiv) floats the idea that the future for producers is to partner with tech savvy start-ups and (xv) describes how US AI supremacy is being challenged. AI: vast and fast growing
It is challenging for baby boomers and older millennials, who populate MedTechs’ C suites, to fully grasp the potential of AI. This is largely because their corporate careers were underway before the digital age started, and for three decades they have personally prospered from manufacturing physical devices without the help of AI. A person who understands the potential of AI is Sundar Pichai, the CEO of Alphabet, one of the world’s largest tech companies. In a recent BBC interview Pichai suggested, "AI is the most profound technology that humanity will ever develop and work on . . . If you think about fire or electricity or the Internet, it's like that, but even more profound". This suggests that Hanson is right to redirect Zimmer’s R&D spend towards AI-driven solutions. A February 2021 report from the International Data Corporation (IDC), a market intelligence firm, suggests that the current global AI market is growing at a compound annual growth rate (CAGR) of ~17% and is projected to reach ~US$554bn by 2024. Data mining, AI, machine learning and neural networks
Among MedTechs’ C suites there is some confusion about data strategies and AI solutions. Many enterprises use data mining techniques on existing large datasets to search for patterns and trends that cannot be found using simple analysis. They employ the outcomes to increase revenues, cut costs, improve customer relationships, reduce risks and more. Although data mining is commonly used when working on AI projects, in of itself, it is not AI. So, let us briefly clarify. AI is the science and engineering of developing intelligent computer programs to enable machines to provide requested information, supply analysis, or trigger events based on findings. AI creates machines that think, learn, and solve problems better and faster than humans. This is different to traditional computing, where coders provide computers with exact inputs, outputs, and logic. By contrast, AI systems can be “schooled” to carry out specific tasks without being programmed to do so. This is referred to as machine learning, which usually requires large amounts of data to train algorithms [mathematical rules to solve recurrent problems]. A critical element of machine learning’s success is neural networks, which is an AI technique modelled on the human brain that is capable of learning and improving over time. Neural networks are comprised of interconnected algorithms that share data and are trained by triaging those data: a process referred to as ‘back propagation’. In healthcare, machine learning outputs range from the ability to recognise images faster and more accurately than health professionals to making in vivo diagnoses. AI systems have become an accepted part of our everyday lives without us realising it
Most people are aware of significant AI breakthroughs such as self-driving cars and IBM’s Watson computer winning the US quiz show Jeopardy by beating two of the best players the show had produced. Lesser known, is in 2012, AlexNet, a neural network learning system, won a large-scale visual recognition contest, which previously was thought too complex for any machine. In 2016, Google’s AlphaGo, a machine learning algorithm, defeated Lee Sedol, who was widely considered the world’s greatest ever player of the ancient Chinese game Go. Most observers believed it would be >10 years before an AI programme would defeat a seasoned Go champion. Although Go’s rules are simple, the game is deceptively complex, significantly more so than chess. It has a staggering 10170 possible moves, which is more than the number of atoms known in the universe. Significantly, machine learning algorithms embedded in AlphaGo, mastered the game without any prior knowledge and without any human input. More recently Google launched AlphaGo Zero, an AI system, which can play random games against itself and learn from it. During the decade of these breakthroughs, AI systems became an accepted part of our everyday lives without us realising it. Examples include, Google searches, GPS navigation, facial recognition, recommendations for products and services, bank loans we receive, insurance premiums we are charged, and chatbots, which organizations use to provide us with information. Technical drivers of AI systems
In addition to commercial drivers, AI techniques are driven by easy availability of data, an explosion in computing power and the increased use of clusters of graphic processing units (GPUs) to train machine-learning systems. These clusters, which are widely available as cloud services over the Internet, facilitate the training of more powerful machine-learning models. An example is Google's Tensor Processing Unit (TPU), which has the capability to carry out more than one hundred thousand trillion floating-point operations per second (100 petaflops). This has the potential to accelerate the rate at which machine-learning models can be trained. Further, the cloud has made data storage and recovery easier, which has motivated government agencies and healthcare institutions to build vast unstructured data sets that they make accessible to researchers throughout the world to stimulate innovation. Obstacles to the development of AI systems
So far, we have emphasised the benefits of AI, but there are concerns that machine intelligence will accelerate at an incomprehensible rate, surpass human intelligence, and transform our reality. This is referred to as “singularity”, which has generated concerns from key opinion leaders. Nearly a decade ago, Stephen Hawking, a pre-eminent British scientist, warned in a BBC interview, that singularity “could spell the end of the human race”. More recently, Hawking’s view has been echoed by Elon Musk, founder, and CEO of Tesla and SpaceX, who suggests that AI is, “more dangerous than nuclear warheads and poses a fundamental risk to the existence of human civilization". Musk has called for stronger regulatory oversight of AI, and more responsible research into mitigating its downsides. In 2015, he set up OpenAI, a non-profit research organization, with a mission to promote and develop AI systems that benefit society. |
|
|
|
|
|
|
|
|
Comments