Directory
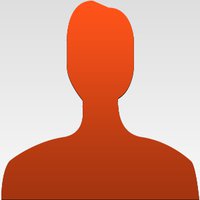
Transforming Healthcare - Top 5 Essential Steps for AI-Based Medical Device Testing
Created by: Qualitest GroupThe disruptive technology Artificial Intelligence is revolutionizing every sector. Especially the healthcare industry. AI-powered medical devices lead to this major change. It drives better patient outcomes and faster diagnostics. It is also redefining patient care. As AI evolves, its impact on medical devices grows. These devices must be safe and effective. Accuracy, safety, and compliance are crucial to their success. Rigorous testing ensures they meet all standards.
This blog covers five key steps to optimize AI-based testing for medical devices. These steps ensure industry standards meet. They safeguard patient trust and deliver measurable value to providers and patients.
1. Focus on data quality and diversity
AI algorithms depend on the diversity and quality of training data. Tips for achieving high-quality data:
Collect data from diverse populations:
This reduces the risk of algorithmic bias. It ensures the device works across different demographics.
Assess data sources:
Collect datasets from reliable and compliant sources.
Preprocess data:
Remove noise, normalize inputs, and address missing values to improve algorithm performance.
Testing teams can improve the reliability of AI-based medical devices. They should focus on data quality. They can also improve fairness by ensuring data diversity.
2. Understand regulatory requirements
Compliance is key to medical device testing. Regulatory organizations like the EMA, FDA, and ISO set strict guidelines. These ensure the safety and efficacy of AI-based medical devices. These regulations cover data quality, algorithm performance, and risk management. Understanding these frameworks is essential before initiating the testing process.
International Organization for Standardization standards:
Global standards define quality management systems. ISO standards provide a clear blueprint for implementation.
Complying with data privacy laws:
Data privacy laws - HIPAA and GDPR ensure the protection of patient data and compliance.
Understanding these requirements ensures the testing process aligns with global standards. It reduces the risk of compliance issues.
3. Develop a robust test strategy
A well-defined test strategy identifies risks in AI-based medical devices. It helps mitigate those risks. This strategy should address various testing parameters, including:
Functional testing:
Ensures the device performs its intended functions well.
Performance testing:
Measures how the AI algorithms analyze data under different conditions.
c) Usability testing:
Validates the user interface. Ensures healthcare professionals and patients use the device with safety and precision.
d) Security testing:
Identifies vulnerabilities to safeguard patient data and prevent breaches.
Diverse testing scenarios replicate real-world conditions. These tests ensure the device performs well in different healthcare environments.
4. Adopt continuous monitoring and validation
Unlike traditional software, AI models evolve over time as they process new data. This requires ongoing validation and monitoring to ensure consistent performance.
Key steps in continuous validation:
Model revalidation:
Assess the AI algorithm to ensure it continues to meet accuracy benchmarks.
Real-time monitoring:
Use analytics to detect anomalies in the device's operation.
Feedback loops:
Incorporate user feedback to refine and improve device functionality.
Continuous validation enhances the device's reliability. It also builds trust among healthcare professionals and patients.
5. Leverage automation and AI-driven testing tools
AI-based medical devices are too complex for manual testing. Automated testing tools enhance accuracy and speed up the process.
Benefits of automation:
Speed:
Automated tests can process large datasets, reducing time-to-market.
Consistency:
Automated tools reduce human error, ensuring repeatable and reliable results.
Scalability:
AI-driven tools can simulate diverse scenarios, enabling comprehensive testing across various conditions.
Popular tools include the TensorFlow Testing Framework and PyTorch. These tools help testers assess AI models. They make the process more efficient. Integrating these tools into the testing workflow ensures thorough evaluation and faster deployment.
Trends in medical devices with artificial intelligence
AI is driving groundbreaking innovations in medical devices, redefining how healthcare delivers. Some notable trends include:
Predictive diagnostics:
AI-enabled devices forecast potential health issues by analyzing patient data. This allows for early intervention.
Remote patient monitoring:
Wearable devices with AI algorithms track real-time health metrics, reducing hospital visits.
Personalized medicine:
AI-powered devices help customize treatments based on individual patient profiles, improving outcomes.
Surgical Support:
AI-guided robotic systems enhance precision in complex surgeries, minimizing risks and recovery times.
These advancements highlight the need for robust testing. It ensures these devices function and remain secure.
Key challenges in testing AI medical devices
Testing AI-driven medical devices presents unique challenges.
Key challenges include:
Algorithm transparency:
AI models, especially deep learning, function as "black boxes." This makes it hard to interpret decisions.
Data bias:
Inadequate or non-representative data can lead to biased outcomes, impacting device reliability.
c) Dynamic updates:
Continuous learning models need constant revalidation to ensure accuracy and compliance.
d) Interoperability:
AI devices must integrate with existing healthcare systems without compromising performance.
Innovative testing strategies address these challenges. They ensure AI-based devices meet the demands of modern healthcare.
Ethical considerations in AI-based testing for medical devices
AI integration in medical devices raises ethical questions. Addressing these questions ensures trust and safety. Key ethical considerations include:
Algorithmic bias:
Ensure AI systems are free from biases. This prevents unequal treatment or outcomes for certain patient groups.
Data privacy:
Protecting sensitive patient data used for training and testing AI algorithms.
Informed consent:
Educate patients and healthcare providers about the role of AI in medical devices. Ensure they understand its limitations.
Accountability:
Establishing clear guidelines for accountability in case of device malfunction or incorrect predictions.
Addressing ethical concerns improves the reliability of AI-based medical devices. It also fosters trust among stakeholders.
How is AI used in medical devices?
Medical devices integrate AI to improve their functionality and efficiency. Harnessing ML and data analytics, AI-powered medical devices provide insights and automate tasks. It enhances the speed and accuracy of medical devices.
Here are some of the key applications:
Image analysis:
AI in radiology devices identify anomalies in MRIs, X-rays, and CT scans. They improve diagnostic accuracy.
Drug delivery systems:
Using AI, smart infusion pumps calculate precise medication dosages, reducing human error.
Predictive health monitoring:
AI in wearable devices detects early signs of conditions like arrhythmias. This enables timely medical intervention.
Virtual health assistants:
AI chatbots provide patients with real-time health advice.
Conclusion
AI based medical devices are transforming healthcare. They provide innovative solutions to longstanding challenges. The safety and efficacy of these devices depend on rigorous testing. Medical device testing ensures they meet the necessary standards. Organizations can understand regulatory requirements. They can develop a robust testing strategy. They can focus on data quality and adopt continuous validation. Automation helps ensure devices meet the highest standards.
Transforming healthcare begins with reliable AI-based solutions. Stakeholders can enhance patient care. They can also adhere to industry standards.
Optimize your testing strategy with Qualitest. Their expert team will help you meet industry standards and improve patient outcomes. Speak with an expert today. They will ensure the safety and compliance of your AI-based medical devices.
go to cluster
Directory:
Tags:
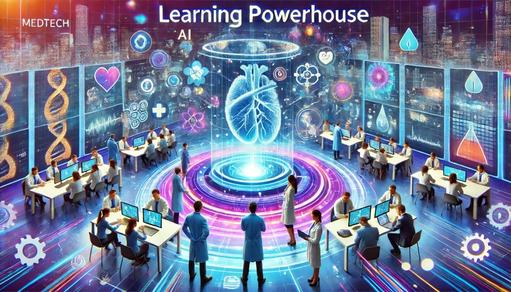
|
|
|



In this episode, we explore how healthcare and MedTech companies can strengthen their resilience in the face of global crises. Rather than relying on reactive strategies, the conversation suggests why proactive, forward-thinking preparation is critical for survival and growth.
Macroeconomic shocks - ranging from AI-driven disruptions and pandemics to geopolitical instability and climate-related challenges - are reshaping the landscape of healthcare and MedTech. This episode unpacks the tools and strategies leaders need to navigate these turbulent times, including supply chain diversification, robust intellectual property protection, and adaptive leadership.
In a world defined by uncertainty, the question isn’t if the next shock will come, but when. Are you ready to face what’s next?
Directory:
Tags:
|
|
|
Directory:
Tags:
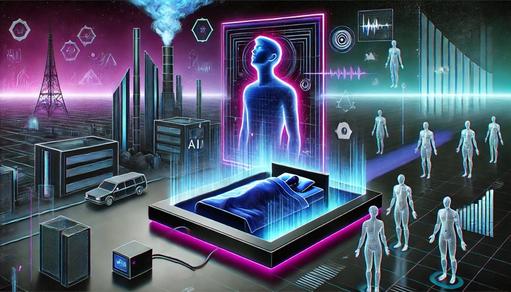
|
|
|
Directory:
Tags:
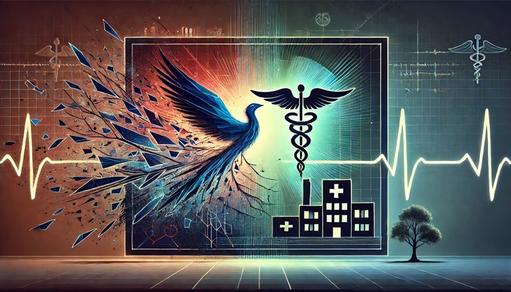
|
|
|
Directory:
Tags:
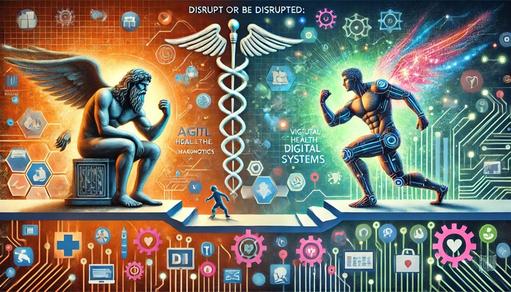
|
|
|
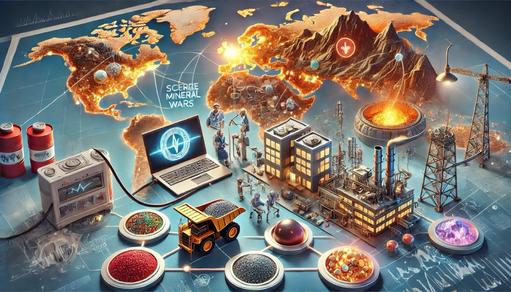
|
|
|